AI-Powered Clinical Data Validation: Ensuring Accuracy, Efficiency, and Compliance
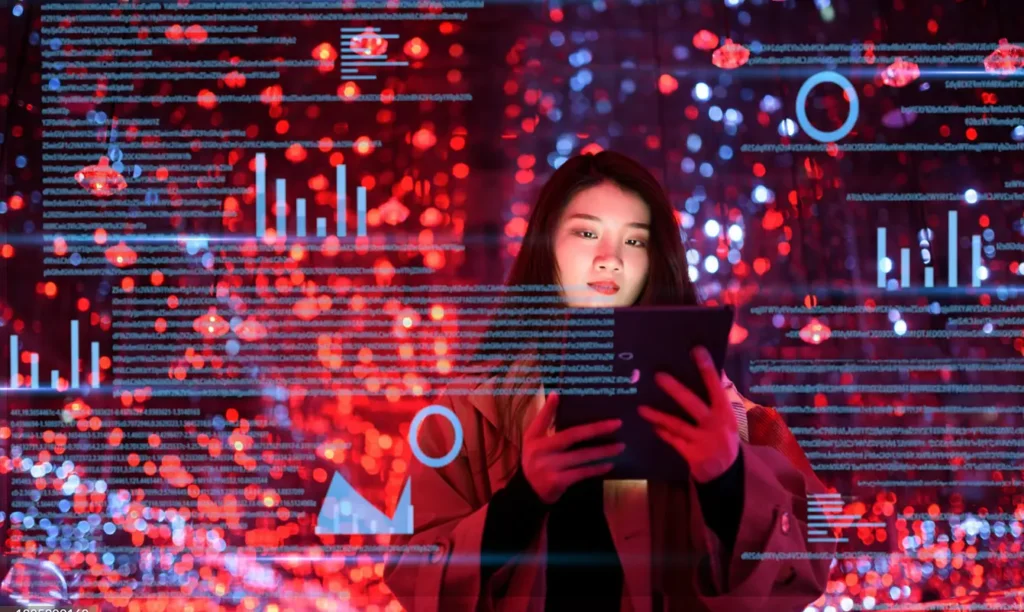
Recent Articles AI in Clinical Trials: Key to Accelerated Timelines & Reduced Costs July 29, 2024 Read More Simplifying eCRFs: A Look at the Future of Clinical Data Management July 8, 2024 Read More AI-Powered Clinical Data Validation: Ensuring Accuracy, Efficiency, and Compliance September 21, 2024 Read More AI-Powered Clinical Data Validation: Ensuring Accuracy, Efficiency, and Compliance Manuj Vangipurapu / September 11, 2024 In today’s complex clinical trial landscape, maintaining data integrity is paramount, as it underpins scientific discoveries and patient safety. With increasing data volumes and trial complexities, traditional validation methods are falling short. AI is emerging as a powerful solution in this context. The Importance of Data Validation in Clinical Trials Data validation is essential in clinical trials to ensure that collected data is accurate, consistent, complete, and reliable through rigorous checks and procedures. As trials become more complex and data-intensive, traditional methods fall short and tend to be expensive and time-consuming. AI tools enhance the efficiency and effectiveness of validation tasks, employing advanced methods to ensure that collected data is accurate, consistent, complete, and reliable. AI-Driven Accuracy Verification: Automating SDV for Precision AI-driven tools improve accuracy verification by automating Source Data Verification (SDV) procedures. Machine learning (ML) algorithms compare electronic data with source documents, detecting discrepancies that manual reviews might miss. AI can play a crucial role in clinical trials for new treatments and medications by analyzing and cross-referencing data from multiple sources, including patient records, clinical trial databases, and historical research studies. AI systems can also accurately integrate data from disparate sources, such as electronic health records (EHRs), laboratory results, and devices. If discrepancies arise, such as differences between lab results and EHR entries, AI flags these for further review. Advanced Consistency Checks with AI: Reducing Errors in Clinical Trials AI algorithms advance consistency checks by analyzing large datasets to uncover patterns and inconsistencies. Machine learning models efficiently identify logical contradictions and discrepancies across records and datasets, providing real-time feedback and alerts when data deviates from expected norms. For instance, AI monitors laboratory test results like blood glucose levels, which should fall within expected physiological ranges. When a recorded value is within range but against the trend, it may indicate a data entry error or instrument discrepancy. AI can apply dynamic thresholds learned from historical data to improve anomaly detection and reduce false positives. Additionally, AI can also be used for complex cross-referencing such as BMI to glucose values which traditional systems do not, and helps create comprehensive risk profiles. AI tools automatically compute derived metrics and cross-check them against recorded data to highlight discrepancies efficiently. Ensuring Data Completeness with AI-Driven Automation AI enhances data quality by not only detecting incomplete or inconsistent information, but also by actively ensuring data completeness. Unlike traditional systems that merely flag missing data, AI can pinpoint gaps and help fill them, ensuring that all required information is documented as per the study protocol. Additionally, by analyzing trends and historical patterns, AI can identify frequently misinterpreted or inaccurately entered fields and offer prompts and assistance through automated bots to improve data accuracy. During the drug development process, clinical trial platforms utilize AI algorithms to continuously monitor incoming data from trial sites, flagging any inconsistencies for immediate review. AI models enhance this by predicting potential data quality issues based on historical trial data. For instance, if certain data fields have historically exhibited higher error rates, the system proactively alerts researchers to focus on these areas. This reduces the need for manual oversight and maintains the integrity of clinical trial results. Advanced AI Techniques for Data Security in Clinical Trials AI strengthens data integrity through cutting-edge security measures. It continually monitors user access patterns and detects any unusual changes. AI can be used to identify unauthorized access attempts to patient data in clinical trial facilities. For example, if a user suddenly tries to access large volumes of sensitive information, AI can immediately trigger additional authentication steps or restrict access to prevent breaches and can also send alerts. After addressing the immediate threat, AI can perform an analysis to assess the breach’s scope, demonstrating its critical role in safeguarding sensitive clinical information. By learning normal user behavior, AI can swiftly identify deviations and flag suspicious activities, effectively protecting against unauthorized access and insider threats. Automating Data Reconciliation and Statistical Review for Faster Insights AI greatly improves data reconciliation by automating the process of aligning information from multiple sources and swiftly addressing discrepancies. Its sophisticated algorithms simplify statistical review by quickly detecting anomalies, outliers, and irregular patterns with high precision. Machine learning models provide advanced insights into data quality and consistency, leading to more dependable statistical evaluations and stronger data integrity. In an oncology clinical trial, an AI system integrated and analyzed data from EHRs and imaging databases, enhancing the statistical evaluation of cancer detection. The AI system increased the accuracy of identifying clinically significant lesions by 20% relative to traditional methods. During pilot studies, the system addressed 10,000 discrepancies in imaging and diagnostic outcomes, and cut data review time by 50%, enabling oncologists to concentrate on more critical tasks. Driving Clinical Research Forward with AI AI has become essential for advancing clinical research. By meticulously safeguarding data integrity, AI enables us to perform trials with exceptional accuracy and dependability. Its ability to automate routine processes, identify anomalies, and foresee potential problems is revolutionizing our approach to clinical studies. As we work toward developing safer and more effective treatments, AI is not just an added benefit but a crucial necessity. Experience the Future of Data Validation with Clinion’s AI Innovations Clinion harnesses AIML and Generative AI to advance data validation in clinical trials, enhancing efficiency, accuracy, and insights. Our AI-driven platform integrates various data sources to ensure consistency and reliability. By enabling intelligent automation, Clinion not only shortens trial timelines but also upholds rigorous data integrity. Our advanced AI, ML, and GenAI modules streamline data validation, boost compliance, and reduce costs, making trial management both seamless and efficient. Get in touch with our product
AI in Clinical Trials: Key to Accelerated Timelines & Reduced Costs
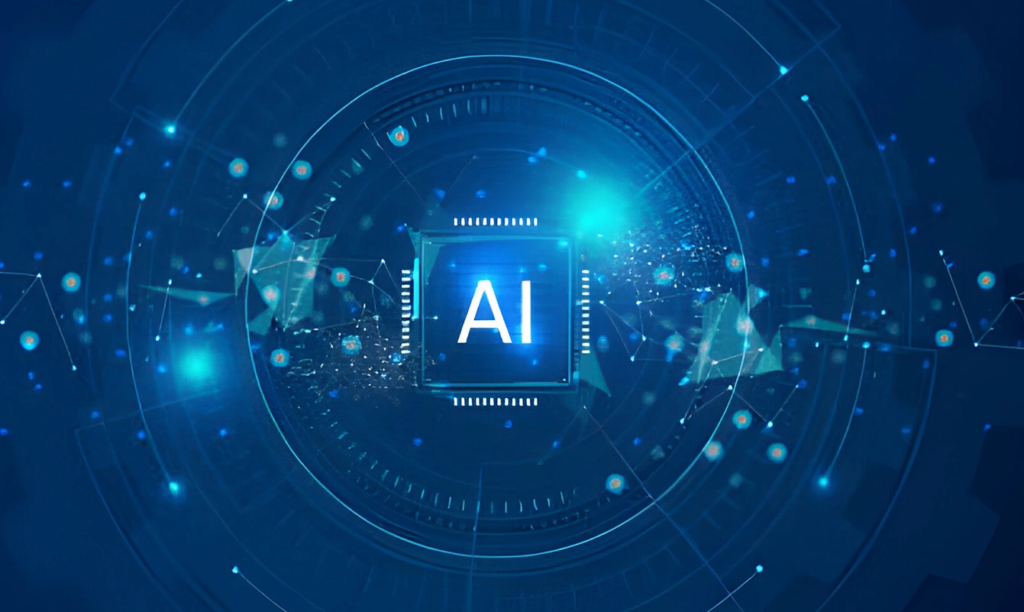
Recent Articles Simplifying eCRFs: A Look at the Future of Clinical Data Management July 8, 2024 Read More AI-Powered Clinical Data Validation: Ensuring Accuracy, Efficiency, and Compliance September 21, 2024 Read More AI in Clinical Trials: Key to Accelerated Timelines & Reduced Costs July 29, 2024 Read More AI in Clinical Trials: Key to Accelerated Timelines & Reduced Costs Tarannum Ara / July 29, 2024 Bringing innovative therapies to patients is a complex and time-consuming process. However, Clinical trials are getting notoriously more time-consuming and costly. But artificial intelligence (AI) is changing the game, offering a promising solution to these age-old clinical trial challenges. A recent study by Nature Digital Medicine revealed that AI-powered patient recruitment can slash clinical trial costs by 70% and expedite timelines by up to 40%. Beyond recruitment, AI is transforming clinical data management, driving faster trial completion and quicker access to life-changing therapies. Overcoming Inefficiencies with AI in Clinical Trials Inefficiencies in data management are a significant hurdle in clinical trials, leading to costly delays. The manual analysis of vast amounts of clinical data is not only time-consuming but also prone to errors. This is where AI comes into play, transforming data management and streamlining the entire trial process. Accelerating Study Setup AI significantly accelerates the study setup phase, reducing months to days. For example, AI automates tasks such as protocol writing and identifying suitable demographics for trials. From creating custom CRFs to analyzing existing ones, AI efficiently handles various aspects of initial study setup requirements. Enhancing Predictive Analytics in Clinical Trials Predictive analytics helps to make real-time decisions during the execution of clinical trials. It can study the pattern of these roadblocks and predict their occurrence in the future. This helps in mitigation of most risks or side effects and allows stakeholders to plan recruitment and trial design according to the prediction, preventing expensive delays and mid-study changes. Revolutionizing Clinical Trial Medical Coding With AI Medical coding is a crucial part of clinical trials and is a time-consuming process. Enter AI, and now hours worth of coding can now be accomplished in a matter of minutes. AI medical coding algorithms can also be trained with millions of biomedical terms for medical context learning using machine learning models. Automating Remote Source Data Verification (rSDV) One of AI’s biggest contributions to clinical trials is remote Source Data Verification. Site monitoring is an expensive and time consuming process. CRAs can now verify source data without travelling to the sites. Sites can upload source data to the AI engine, which extracts this data and compares it with data entered into the EDC. Matched data items are marked as ‘SDV’. The system automatically generates queries on unmatched data and assigns to sites. Harmonizing Real World Data (RWD) in Clinical Trials AI plays a crucial role in the analysis of Real World Data (RWD) and harmonization of data. With the growing importance of RWD in clinical trials, the harmonization of the same is also becoming a need of the hour. Artificial intelligence automates processes like data cleaning and offers next gen features like NLP and pattern recognition which helps in making most of the large data sets that are created in the process of clinical trials. Beyond Cost-Cutting: Additional Benefits of AI in Clinical Trials The implementation of AI in clinical trials is not only reducing the cost; it has its impact on many other areas of clinical trials as well. From optimizing drug doses to identifying treatment protocols, AI has changed the clinical trial scene positively. With reduced errors and personalized treatment allocation to patients, AI contributes to accelerated trials at reduced cost. Clinion is the industry’s first AI-enabled eClinical platform that ensures faster, accurate and affordable clinical trials ensuring life-saving treatments reach the patients quickly. Our platform is a powerful amalgamation of technology and healthcare promising a revolution in the life science segment. Get in touch with our product experts today to learn more Contact Us
Simplifying eCRFs: A Look at the Future of Clinical Data Management
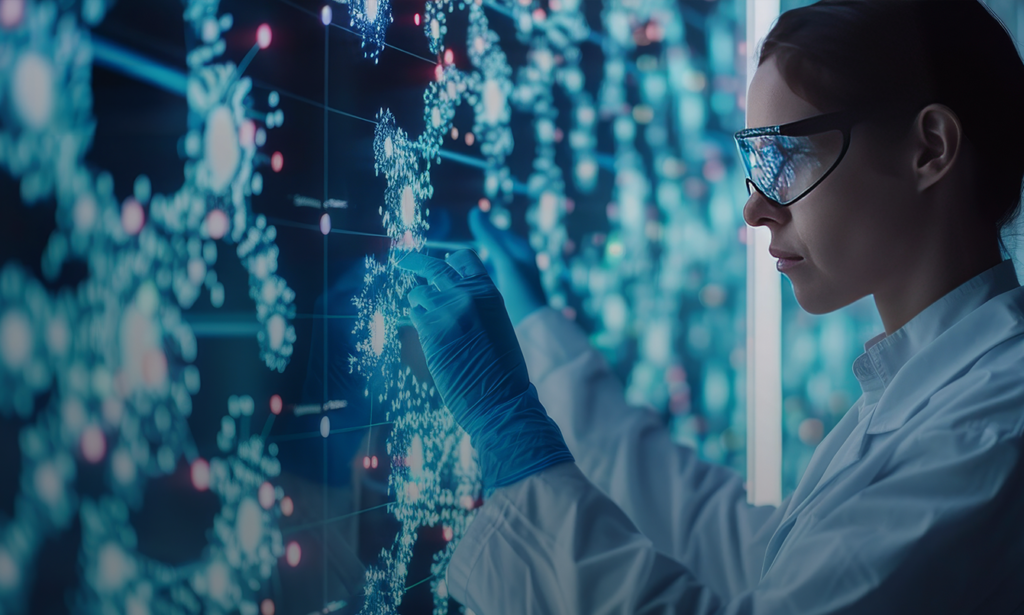
Recent Articles AI-Powered Clinical Data Validation: Ensuring Accuracy, Efficiency, and Compliance September 21, 2024 Read More AI in Clinical Trials: Key to Accelerated Timelines & Reduced Costs July 29, 2024 Read More Simplifying eCRFs: A Look at the Future of Clinical Data Management July 8, 2024 Read More Simplifying eCRFs: A Look at the Future of Clinical Data Management Abriti Rai / July 08, 2024 The evolution of electronic case report forms (eCRF) is a remarkable progress in the clinical trials landscape. The transition from paper-based CRFs to eCRFs was driven by several factors, including the increasing complexity of clinical trial protocols, the need for greater efficiency in managing large volumes of trial data, and advancements in technology that supported digital solutions for data collection and management. The main objective of this shift was to obtain accuracy and speed in data collection, data validation, enhanced security, and the overall improvement of the clinical trials. As electronic case report forms (eCRFs) have continued to advance, they have adapted to the evolving trial requirements and contributed to the standardization of the data collection process. The Journey from Paper to Electronic Case Report Form (eCRF) Paper-based electronic case report forms (eCRF) need manual data entry, which often leads to transcription mistakes and illegible handwriting, compromising data quality. The need for physical transportation of forms caused significant delays in data processing, hindering timely decision-making. Managing paper forms is labor-intensive and resource-consuming, and they are vulnerable to damage, theft, and unauthorized access. In contrast, eCRFs bring numerous benefits and are the answer to the challenges faced by paper case report forms (CRFs). Built-in validation checks and standardized input fields reduce errors, ensuring more accurate data collection. Instant data entry and real-time access improve decision-making and trial efficiency. The elimination of paper reduces administrative costs and resource use. eCRFs ensure standardized data collection and regulatory compliance by incorporating various security measures such as encryption, role-based access control, and multifactor authentication to protect data integrity and confidentiality. Additionally, robust disaster recovery strategies are implemented to safeguard against data loss, which are essential features of modern eClinical systems. The Rise of Global Libraries and Built-in Compliance With the shift towards eCRFs, the industry is also embracing pre-built global libraries that streamline data collection, ensure regulatory compliance from the outset, and contain standardized data points adhering to CDASH guidelines. This eliminates the need to recreate forms from scratch for each study, saving time, ensuring consistency across trials, and expediting study initiation with compliance built right in. Gen AI and AIML Shaping the Future of eCRFs As mentioned before, Case report forms (CRFs) have evolved from paper forms to electronic forms to meet the changing needs of a clinical trial. But their transformation is still ongoing and the incorporation of Generative AI (Gen AI) and Artificial Intelligence/Machine Learning (AIML) plays a pivotal and game-changing role in further revolutionizing the effectiveness of electronic case report forms (eCRFs). The incorporation of AI and ML into eCRFs promises efficient and informative trials, reducing costs, expediting timelines, and ultimately increasing the likelihood of successful outcomes. Here’s a look at some exciting advancements on the horizon: Automate eCRF Creation GenAI streamlines electronic case report form (eCRF) creation by intelligently analyzing clinical trial protocols, leveraging pre-built global libraries of standardized data fields, and automatically drafting eCRFs. This reduces manual effort, minimizes errors, and saves time. Mapping of CDASH Annotations CDASH is a part of the CDISC initiative and guides eCRF development. By mapping CDASH annotations in eCRFs, researchers can ensure that the data is captured in the eCRFs with standardized CDASH terminologies. This, in turn, standardizes the collection, documentation, and reporting of the data and interoperability, thereby facilitating data sharing and regulatory compliance across the research community. Source Data Verification (SDV) AIML can be used in the electronic case report form (eCRF) system to ensure that the data collected for the trial is valid. Using AIML, the information collected from the participants for the clinical trials can be compared to the actual source. Any anomalies or inconsistencies in the data can then be detected. SDV is instrumental in upholding good clinical practice (GCP), which is extremely important for clinical research. Automate Query Generation The integration of Al with eCRFs helps in the detection of anomalies. However, it also automates query generation through alerts and notifications to the concerned personnel, which helps streamline the query resolution process and the optimization of trials. Transformative Leap of Electronic Case Report Forms (eCRFs) Integration with technologies such as Gen AI and AIML is leading us toward a new era of smart eCRFs to automate form creation, standardize data collection, verify data sources, and automate query generation. This demonstrates the potential that technology holds in influencing the future of data collection in clinical trials that translates to faster study setup, cleaner data, and ultimately, getting new treatments to patients quicker. Clinion: Advancing Streamlined eCRFs At Clinion, we are at the forefront of the eCRF revolution. We continuously innovate and develop new tools that leverage cutting-edge technology like global libraries, AIML, and GenAI. Our mission is to empower researchers with smarter electronic case report forms (eCRFs) that accelerate the development of life-saving treatments. Get in touch with our product experts today to learn more.